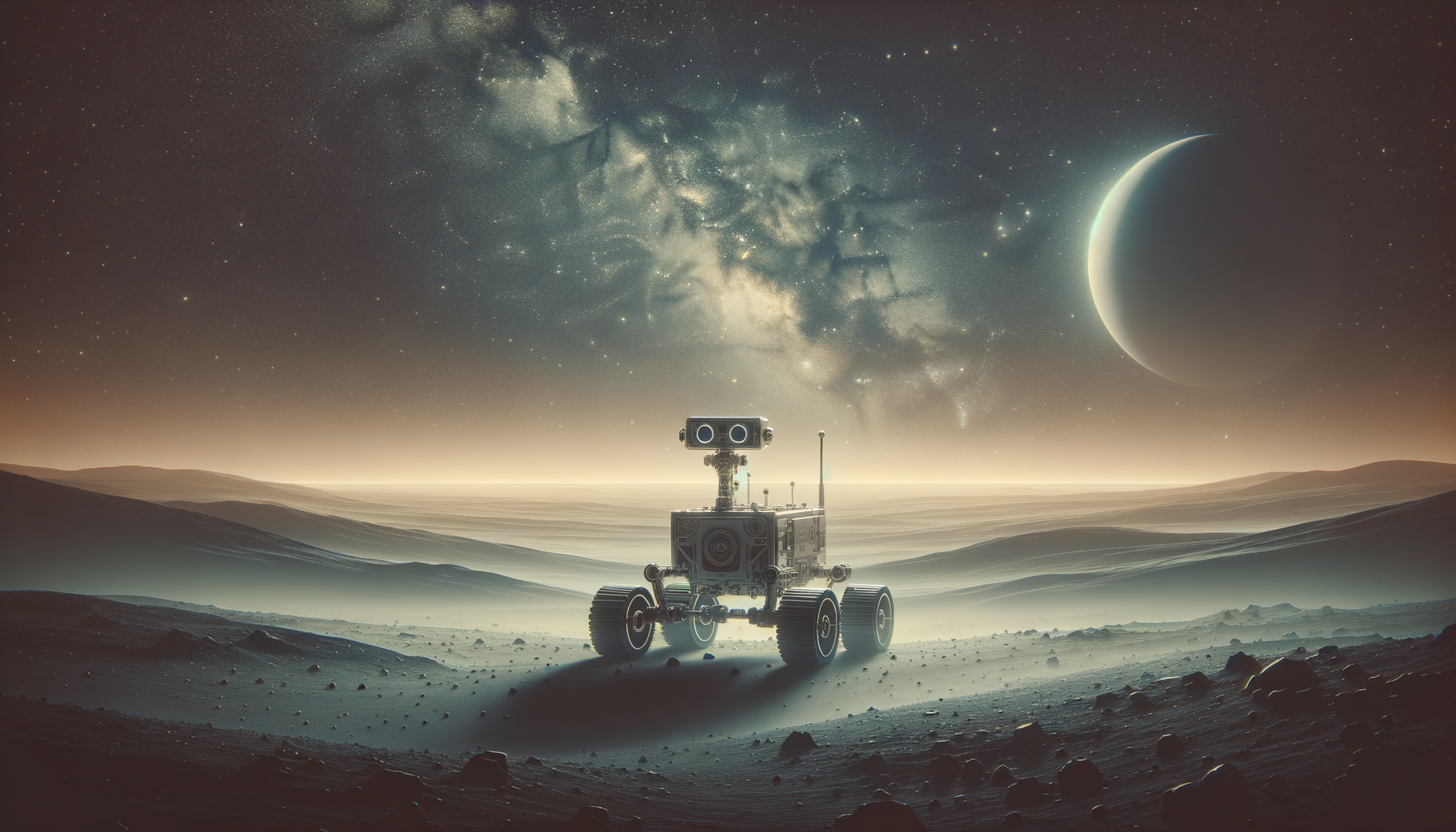
Predict. Adapt. Protect.
StarBayes transforms cost-effective hardware & robotics into cognitive agents that predict, adapt, and exploit their surroundings in ways that overpower traditional Deep Learning solutions.
Environment-Aware Robotics, Made Affordable
StarBayes turns low-cost drones, wheeled robots, rovers and future satellites into cognitive agents. Running on Raspberry Pi-class hardware, each unit builds a hierarchical Bayesian world-model of its surroundings, prioritises actions with a need-based attention layer, and uses embedded tools to convert predictions into motion. Because decisions are computed on-board—then handed off via solar relay pads for 24 / 7 coverage—the robots adapt in ways pre-trained deep-learning systems cannot, by using their environment and solving uncertainties from sheltering under a branch before rain to reinforcing a dune before a surge.
GeoVisor — Forest & Ecosystem Edition
-
Forestry agencies, conservation NGOs and private-estate owners need continuous, on-site intelligence to prevent wildfires and biodiversity loss. A ten-to-fifteen-unit GeoVisor fleet rotates through a solar relay pad, so at least one drone or rover is always active. The system forecasts fire paths, drought pockets and habitat stress up to 48 h in advance, giving staff the lead time to open firebreaks, adjust irrigation or relocate wildlife—actions that satellite passes or manual patrols cannot deliver in time. Click on the Lern More buttin below for the GeoVisor whitepaper.
-
3-D-printed air and ground frames carry Raspberry Pi brains, depth/LiDAR cameras, temperature-humidity stacks and optional soil-moisture probes. All units dock at a single solar pad for charge and data upload, keeping a 10-hectare block under 24/7 watch without oversized batteries.
-
On-board code builds a vertical-plus-horizontal Bayesian hypergraph that links trees, undergrowth, wind and fuel moisture to their likely futures. Need-based attention raises the priority of “energy” or “fire risk,” while embedded metric tools compute shortest paths—enabling a drone to shelter under a branch it was never explicitly trained to use.
CoastVisor — Shoreline Edition
-
Coastal municipalities, port authorities, insurers and habitat managers face escalating erosion and storm-surge damage. A relay ring lets drones swap eight-hour shifts while rovers adjust sand-fences or smart tide-gates. The system models dune movement, tide cycles and wind fields to predict breach points and flood corridors up to two days ahead, providing asset-level warnings and intervention cues that fixed sensors miss.
-
Pi-powered quadcopters, small tracked rovers and fixed actuator nodes share low-profile solar hubs for charging and data sync. Sensor suites—depth cameras, GPS, barometers, anemometers and soil-moisture pins—remain inexpensive thanks to 3-D-printed mounts and commodity parts.
-
The coastal world-model extends the hierarchical graph to dunes, berms and water levels. Factor-graph inference calculates breach probabilities, and need-driven attention flags rapid moisture loss or wind shifts. Built-in path tools steer rovers to reinforce the exact metre of dune predicted to fail, not just where erosion is already visible. Click the Learn More button below to read the CoastVisor whitepaper.